
Research
Advancing the theory, design, development and adoption of ethical AI systems
Gradient Institute conducts research on responsible and ethical AI, often in collaboration with universities and other research institutions, and shares its findings across the research community through publications, presentations and forums. On this page are some of our areas of focus.
Algorithmic fairness
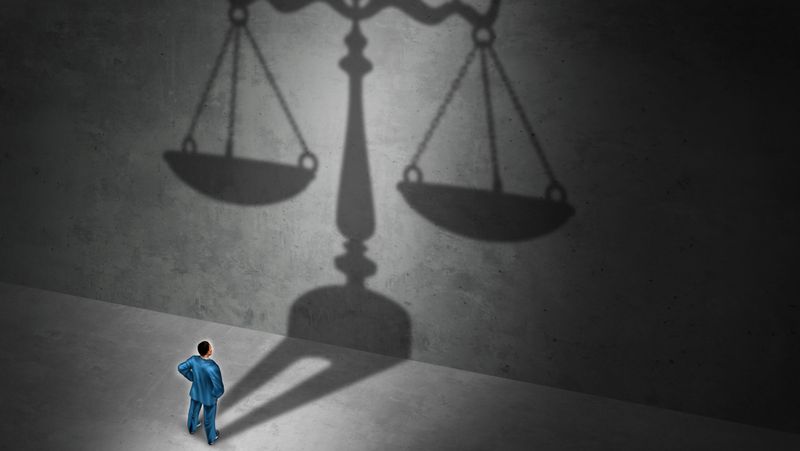
HOW can we ensure AI systems make decisions fairly? Algorithmic fairness involves expressing notions such as equity, equality or reasonable treatment as equations that a machine learning algorithm can seek to optimise as it makes automated decisions.
Turning these ethical concepts into mathematical terms so a computer can understand is a significant challenge that requires a multidisciplinary and multi-stakeholder approach. The same can be said for the need to balance between fairness and other often competing objectives, such as the predictive accuracy of the automated decisions. Our research is driven by case studies, developments in the discipline and partner use examples, and hence has a very applied focus.
Anti-discrimination law
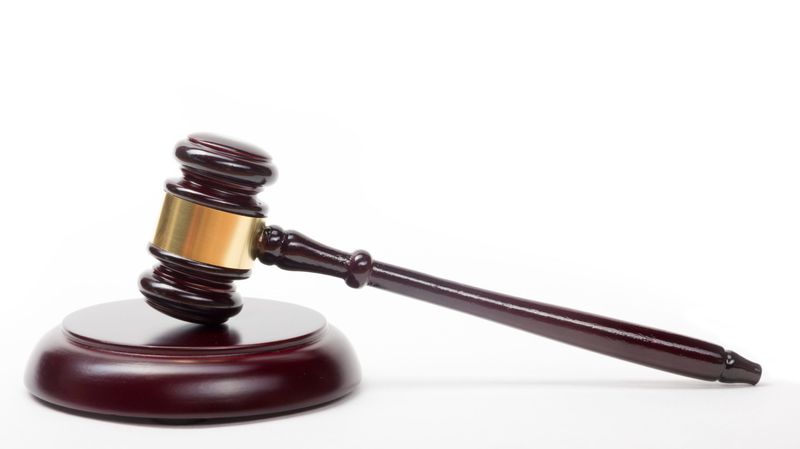
EXISTING anti-discrimination laws have developed on the assumption that decisions about people would be made exclusively by people.
This is no longer the case, as AI systems play a growing role in decisions that affects individuals. And since people and AI systems process information very differently, AI systems need to be adapted to ensure they do not act in violation of existing laws.
We explore how existing legislation can be applied to algorithmic decision-making systems, and whether important gaps exist and how they might be addressed. This work is conducted in collaboration with legal scholars who specialise in anti-discrimination law.
Causality
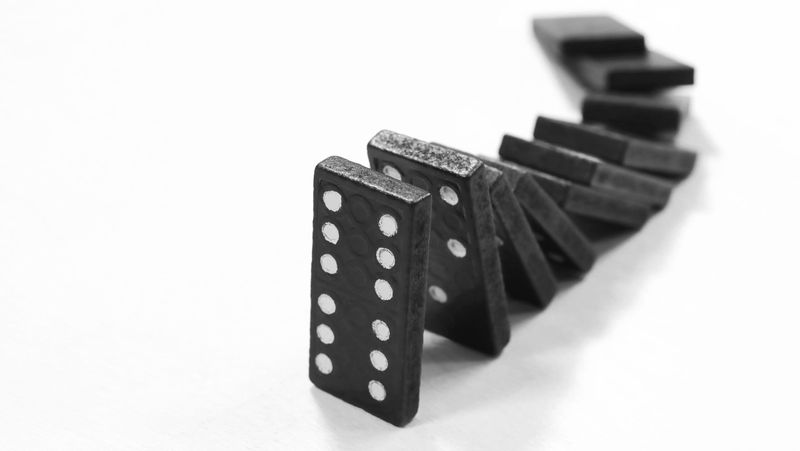
CAUSALITY is 'cause and effect': how an event, process or state influences another – where the cause is partly responsible for the effect, and the effect is partly dependent on the cause.
In AI, causality is mathematically represented, statistically modelled and used to support effective decision-making. In order to ensure AI systems make ethical decisions, we need to understand – at a deep level – how different design choices for a system may affect its decisions and the impact they have on different people.
To do so, we explore the causal relationships that arise from ordered sets of instructions governing how a system transforms data inputs into processed data outputs, as well as the downstream effects a system’s decisions have on people. This research is conducted in collaboration with industry and government partners who are deploying AI systems at scale.
Ethics of insurance
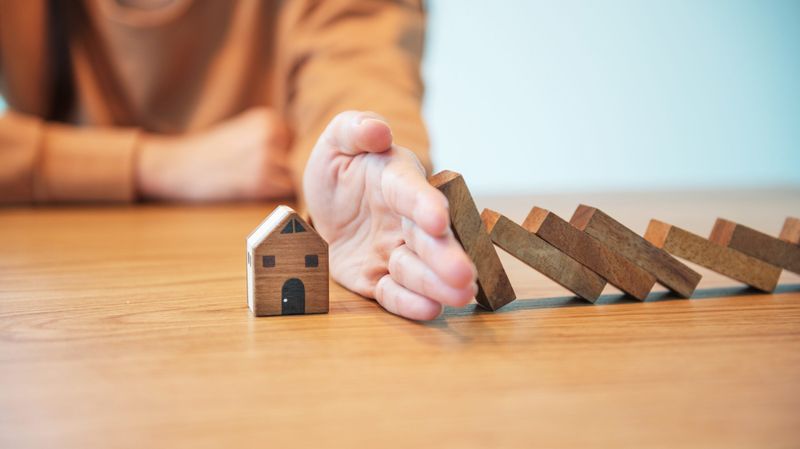
INSURANCE is a fundamental ingredient supporting the smooth functioning of modern societies. Everyone is subject to ‘bad luck’, and insurance is a mechanism to ensure people are compensated when bad luck strikes.
As more data becomes available and AI develops, insurance companies can in principle increase their ability to predict individual risk. If it’s legal for a company to use some data to improve risk estimates, should it always use it? What does “ethical” insurance pricing mean when the data tells us the risks for some people are much higher than for others?
We collaborate with experts in insurance and moral philosophy to investigate the complex ethical questions related to insurance pricing in the age of data and AI.
If you'd like to discuss research collaborations, postdoctoral opportunities, training or other collaborations, please don't hesitate to contact us.