
Training
Understanding the ethical implications of AI in decision-making
Gradient Institute offers training for organisations in responsible AI. Gradient's courses teach how to build, procure, operate and govern AI systems that are safe, beneficial, trustworthy and that avoid unintentional harm. This includes AI systems used for automated decision-making, generative AI and other purposes.
We teach how to align the impact of an organisation's AI systems with its objectives and values.
Gradient's approach to training is driven by personal experience. The core of our courses are interactive discussions and activities that draw from the real problems faced by participants. The courses are led by highly experienced senior researchers at Gradient who have personally faced these same problems.
Our training offerings are specialised across all roles in an organisation: from board-level, through to senior leadership, technical and operational staff. We cater to all levels of knowledge, from staff who are new to AI to researchers with PhDs.
Learn more about our course offerings below, or get in touch to customise a course to your needs:
Responsible AI Primer
Duration: 3 hours (2h abridged variant available)
Audience: For those with no existing knowledge of AI / machine learning (ML)
Format: online or in-person
Topics covered:
- the uses of AI today
- how different kinds of AI work
- AI's opportunities and risks
- when AI should and shouldn't be used
- responsible AI and the Australian AI Ethics Principles
LLM Risk and Controls
Duration: 3 hours (2h abridged variant available)
Audience: Anyone working with large language model systems
Format: online or in-person
Topics covered:
- LLM's increasing relevance and how their algorithms work
- new LLM capabilities and potential applications
- specific risks associated with the deployment of an LLM
- controls for managing and mitigating risks
- changes to the AI lifecycle to support effective LLM governance
Responsible AI Governance
Duration: 3 hours (2h abridged variant available, 1h for board variant)
Audience: For senior staff of the organisation such as those at an executive or general manager level.
Format: online or in-person
Topics covered:
- how AI operates differently from human decision makers
- novel opportunities and risks from AI systems
- gaps in existing governance approaches
- bridges between technical teams and senior decision makers
- appropriate accountability and oversight
Board variant: A shorter version of this course containing a targeted overview of RAI governance concerns. It is focussed on how the use of AI impacts the duties of company boards. Designed for Board members or equivalent senior-level staff.
Legal variant: Gradient Institute also partners with a specialist emerging technology law firm and can provide RAI governance training courses that incorporate technical and legal considerations. These are not described in this document. Please contact us for more information about these courses.
Responsible AI Systems
Duration: 1 day
Audience: AI development team members including data scientists, engineers and product owners
Format: online or in-person
Topics covered:
- foundations of responsible AI
- sources of unintended harm
- definition and measurement of objectives
- fairness and explainability
- risk reduction steps in the AI lifecycle
- accountability, monitoring and review
Recent engagements
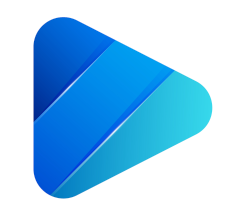
Gradient Institute has provided Responsible/Ethical AI training for many large organisations over the past four years including major banking, insurance, telecommunications and health companies, major digital platforms, leading data analytics companies, and many others.
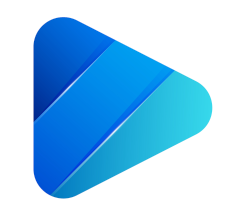
We have also provided training through other organisations to third party organisations and have done so separately for AI startups, medical researchers, healthcare professionals and others.
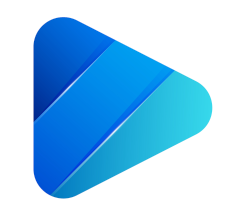
Most of the training has been within Australia but we have also delivered training to organisations overseas (such as Singapore, The Philippines and US).
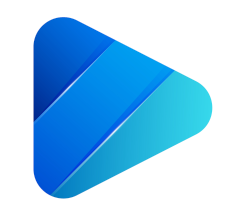
We were approached by the Australian Government to exclusively provide training at their 2021 AI Summit. This was an “AI Primer” designed for a wide range of delegates. It received positive feedback from attendees and the government.
If you would like to enquire about these courses, or about potential customisation, please contact us.