Gradient Institute collaborated with the Australian Capital Territory (ACT) Education Directorate, to apply causal machine learning techniques to investigate drivers of student outcomes at school.
The motivation of this work was to contribute to the Directorate’s plans for improving long-term learning growth and other outcomes for students, including student wellbeing.
Issues arise when using traditional statistical approaches to causal inference on datasets in which there are complex relations, or that have many attributes (columns) relative to the sample size (rows) of the data – as is the case for most modern datasets. This scenario is where machine learning models excel, and by combining the theory from traditional causal inference techniques, with the power of machine learning (see Figure), we can tackle increasingly large and complex causal inference problems.
This project was made possible in part by the world-leading work done by the Directorate and the Australian National University’s Research School of Psychology in collecting a comprehensive set of data about its students and the school system including:
- Background demographic information on students (including but not limited to parent’s education levels and occupations).
- Longitudinal data on students’ wellbeing, including how they identify with their school and engage with their peers, teachers and their schools (in the form of a “climate” survey).
- Teacher experience and shared values, but also how staff identify with their school, and how they interact with one another and with school leadership, their professional development and morale.
To take full advantage of this extensive dataset, researchers at Gradient Institute used state-of-the-art machine learning techniques for causal inference that enabled several highly controlled studies of the data to be conducted. These studies included estimating the, possibly non-linear, effects of school staff and leadership on student wellbeing, as well as on reading and numeracy outcomes (NAPLAN). Also under examination was the effect of student wellbeing on reading and numeracy outcomes. These studies highlighted several interesting and strong relationships that may be the target of the Directorate’s future policy work.
In parallel with this work, the ANU Humanising Machine Intelligence team used insights from various areas of philosophy to identify theoretical questions and frameworks useful for informing work on analysing education data.
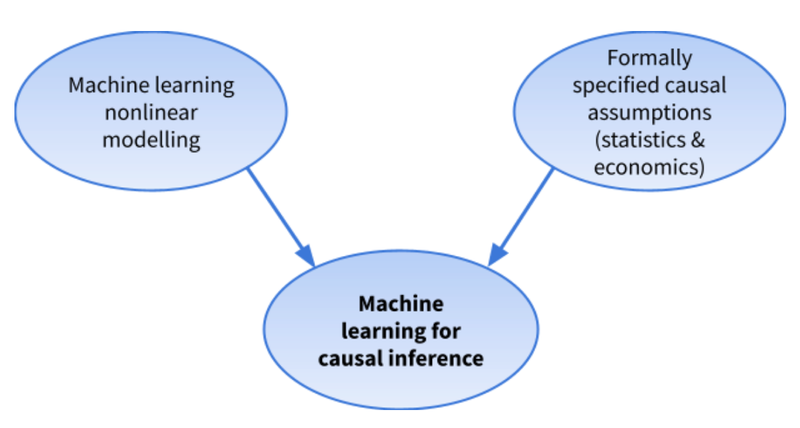
Figure: Causal machine learning techniques combine the powerful modelling ability of machine learning with the rigorous causal inference theory from statistics and economics. This allows for highly controlled studies to be performed, which can also uncover nonlinear causal relationships.