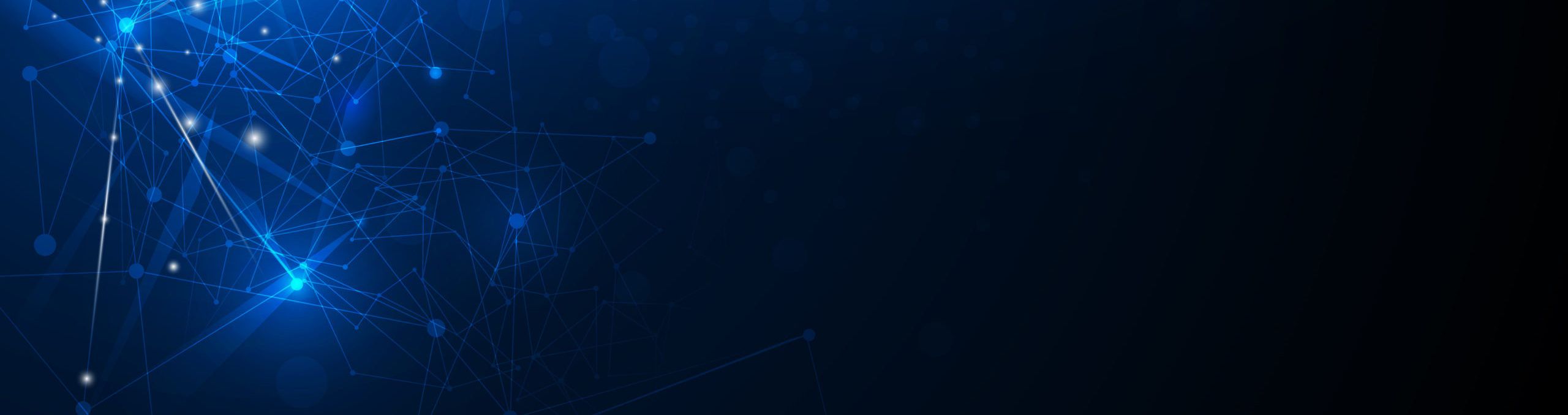
Articles
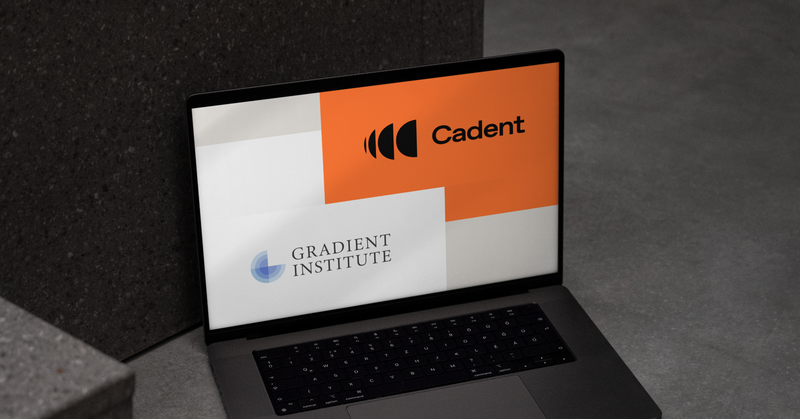
Gradient Institute Receives Donation from Cadent for AI Safety Research
9 November 2023
Gradient Institute has received a donation from Cadent, an ethical technology studio, to develop research on technical AI Safety.
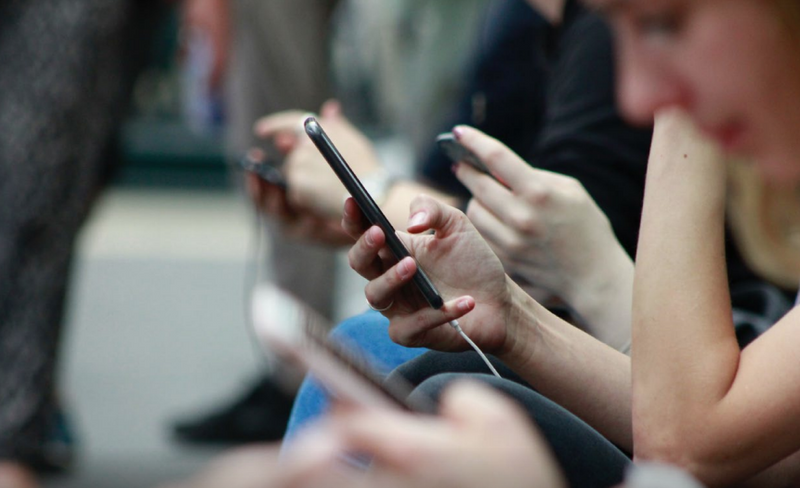
New report to help businesses implement responsible AI
22 June 2023
Gradient Institute, in collaboration with Australia's National Artificial Intelligence Centre (hosted by CSIRO), has released a report that outlines practical measures for businesses to adopt Responsible Artificial Intelligence.
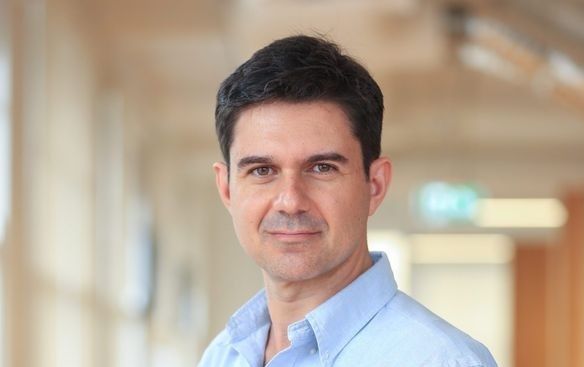
Regulators urged to monitor the AI power struggle closely for potential risks
1 May 2023
Dr. Tiberio Caetano, co-founder and chief scientist of Gradient Institute, warns that regulators need to start paying attention to anyone using vast amounts of computer power in machine learning to prevent “bad actors” from creating harmful new AI systems.
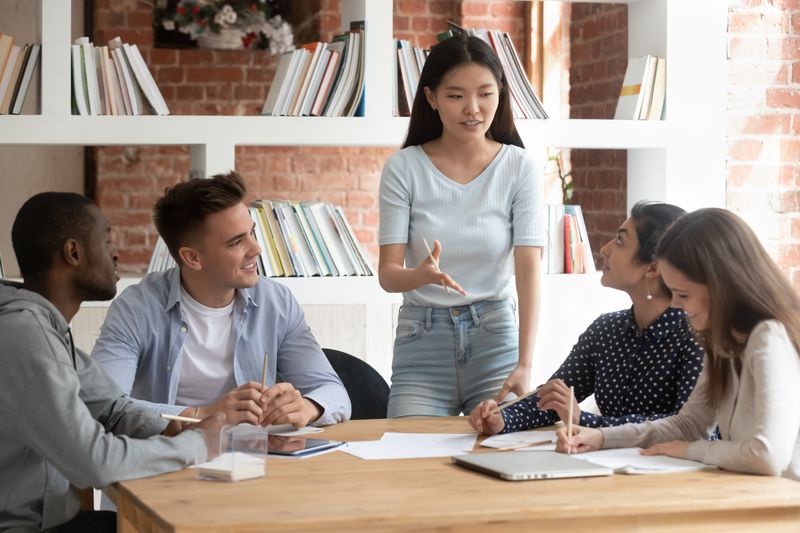
Banking Finance + Oath (BFO) AI-driven marketing in financial services
9 June 2022
The BFO Young Ambassadors collaborated with Gradient Institute to investigate the ethical concerns surrounding the use of AI in financial services. While some areas of the industry, such as credit scoring, have gained awareness of AI ethics, little attention has been given to AI-driven marketing. Developing a thorough understanding of the ethical considerations will help financial services...
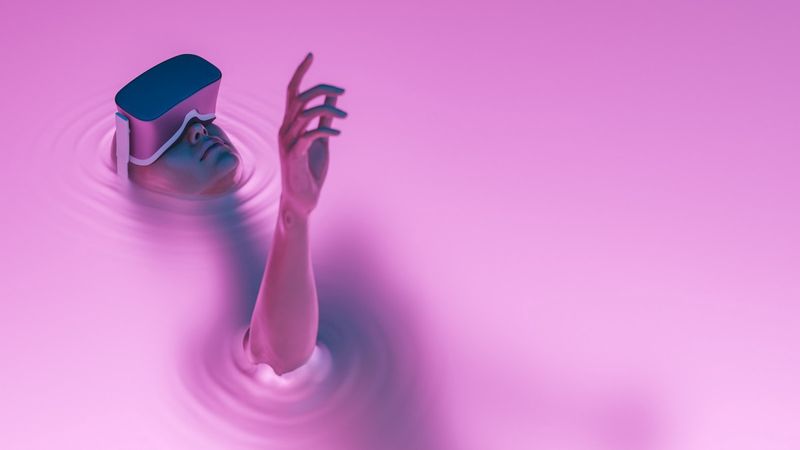
Caution: metaverse ahead
21 April 2022
Gradient Institute’s Chief Practitioner, Lachlan McCalman wrote this latest blog post about the metaverse on Medium. The post argues that the metaverse has the potential to have a profound impact on the world, and as a result, we would be wise to plan conservatively...
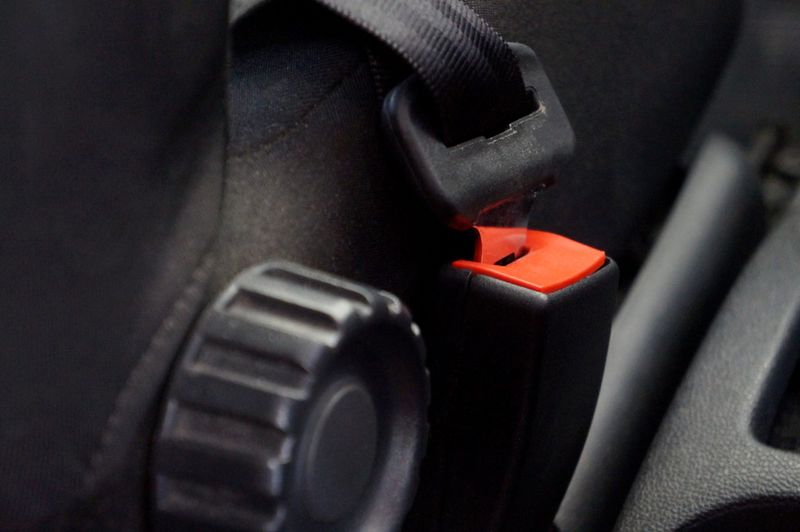
De-risking automated decisions
16 March 2022
Today, in collaboration with Minderoo Foundation, we are releasing a report on de-risking automated decisions, which includes practical guidance for AI governance and AI risk management.
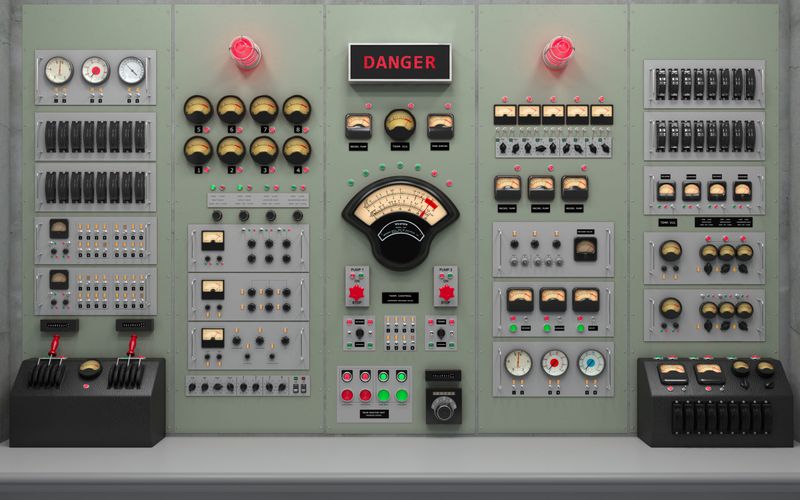
AI Impact Control Panel
16 March 2022
In partnership with Minderoo Foundation, Gradient Institute has released the first version of our AI impact control panel software. This tool helps decision-makers balance and constrain their system’s objectives without having to be ML experts.
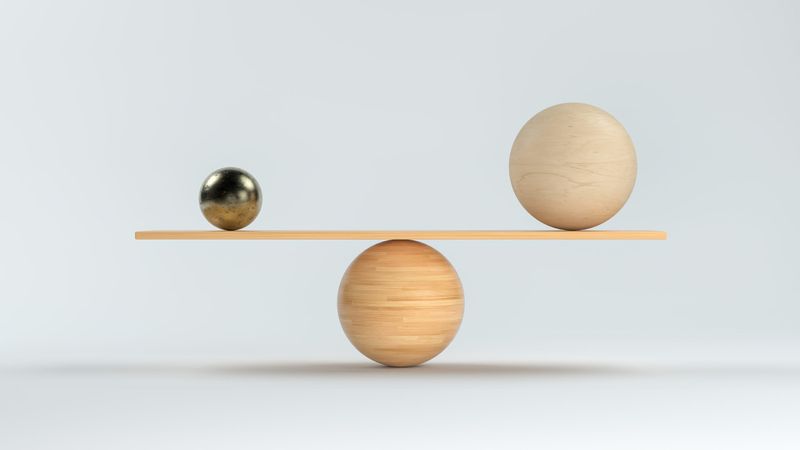
Designing a practical approach to AI fairness
22 February 2022
Gradient Institute, along with collaborators from ServiceNow, Vector Institute and The University of Tübingen, just published an article in the January edition of IEEE Computer laying out conceptual foundations for practical assessment of AI fairness.
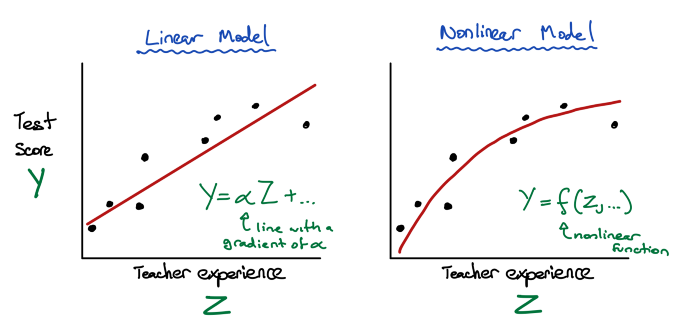
Machine learning as a tool for evidence-based policy
23 July 2021
In this article, Gradient’s Dan Steinberg and Finn Lattimore show how machine learning can be used for evidence-based policy. They show how it can capture complex relationships in data, helping mitigate bias from model mis-specification and how regularisation can lead to better causal estimates.
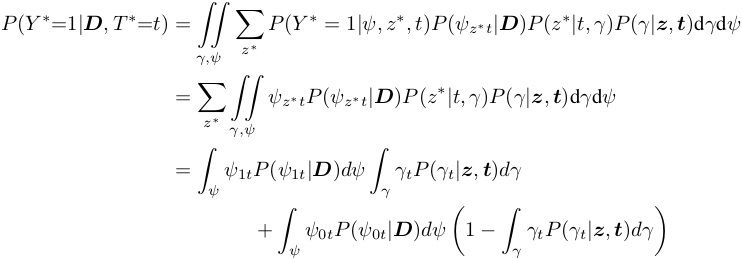
Explainer on Causal Inference with Bayes Rule
27 April 2021
In this post we explain a Bayesian approach to inferring the impact of interventions or actions. We show that representing causality within a standard Bayesian approach softens the boundary between tractable and impossible queries and opens up potential new approaches to causal inference. This post is a detailed but informal presentation of our Arxiv papers: Replacing the do calculus with Bayes...
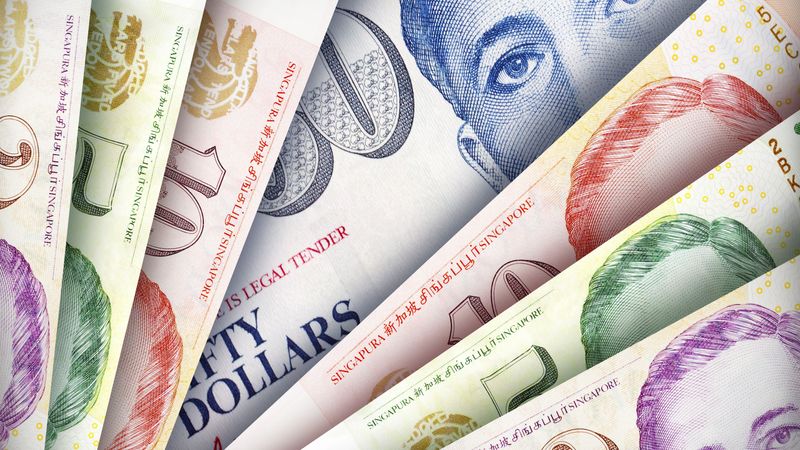
Practical fairness assessment for AI systems in finance
15 April 2021
Gradient Institute’s Chief Practitioner, Lachlan McCalman, describes our collaborative work with the Monetary Authority of Singapore and industry partners to develop a practical AI Fairness assessment methodology.
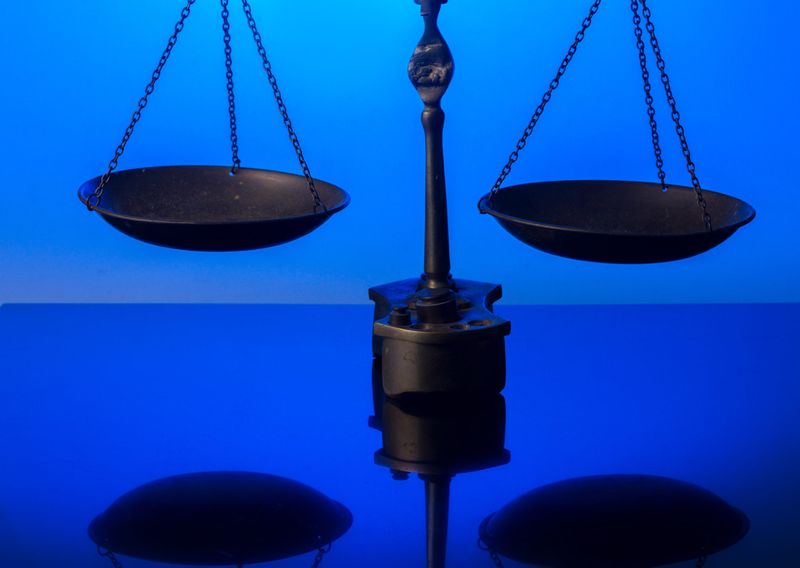
Next-best-action for social good
22 March 2021
Gradient’s Chief Scientist, Tiberio Caetano, explains how next-best-action systems are often used to optimise business metrics and individual customer outcomes, but questions whether they could also become a vehicle for promoting social good.
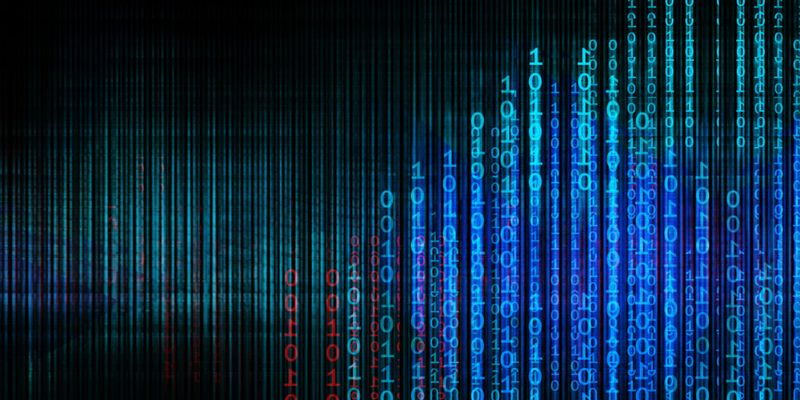
Preventing AI from deepening social inequality
13 January 2021
An article in The Conversation by Gradient’s Tiberio Caetano and Bill Simpson-Young discussing a technical paper co-written with Australian Human Rights Commission, Consumer Policy Research Centre, CHOICE and CSIRO’s Data61.
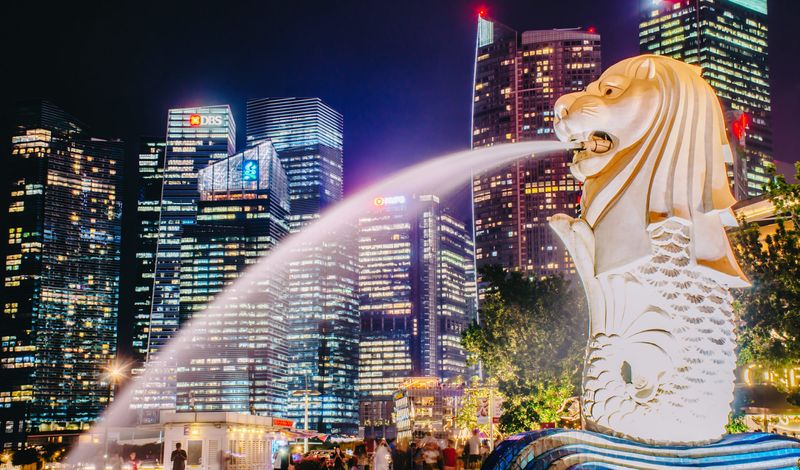
Monetary Authority of Singapore announces success of Veritas Initiative phase 1
6 January 2021
The Monetary Authority of Singapore (MAS) announced the successful conclusion of the first phase of the Veritas initiative which saw the development of the fairness assessment methodology in credit risk scoring and customer marketing. These are the first two use cases to help financial institutions validate the fairness of their Artificial Intelligence and Data Analytics (AIDA) solutions...
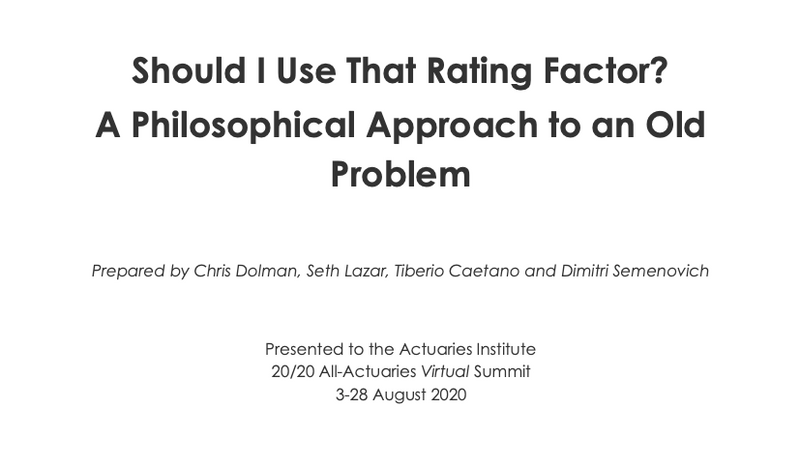
Ethics of insurance pricing
4 August 2020
Gradient Institute Fellows Chris Dolman, Seth Lazar and Dimitri Semenovich, alongside Chief Scientist Tiberio Caetano, have written a paper investigating the question of which data should be used to price insurance policies. The paper argues that even if the use of some “rating factor” is lawful and helps predict risk, there can be legitimate reasons to reject its use. This suggests insurers...
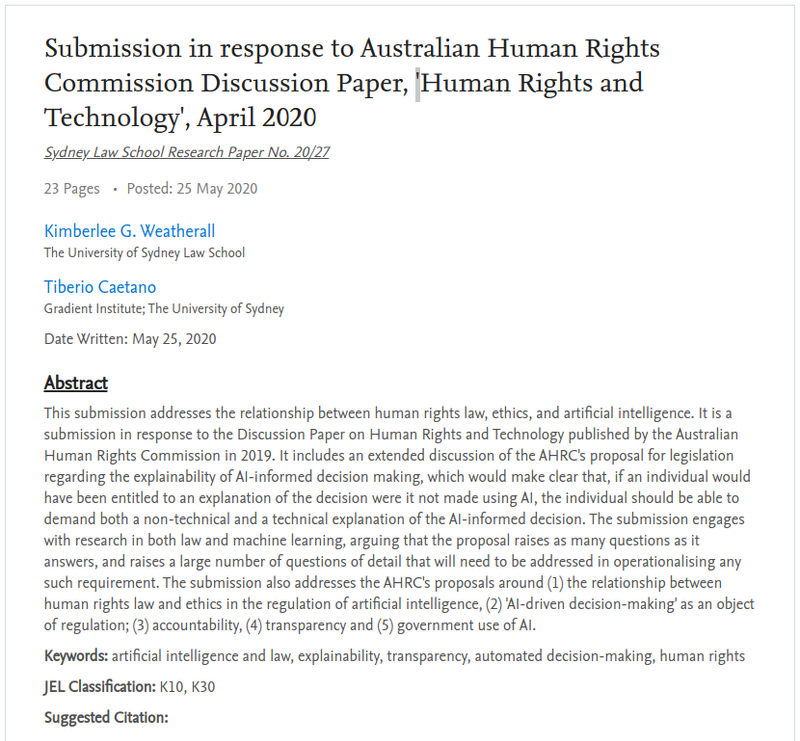
Submission to Australian Human Rights Commission
25 May 2020
Gradient Institute Fellow Kimberlee Weatherall and Chief Scientist Tiberio Caetano have written a submission to the Australian Human Rights Commission on their “Human Rights and Technology” discussion paper.
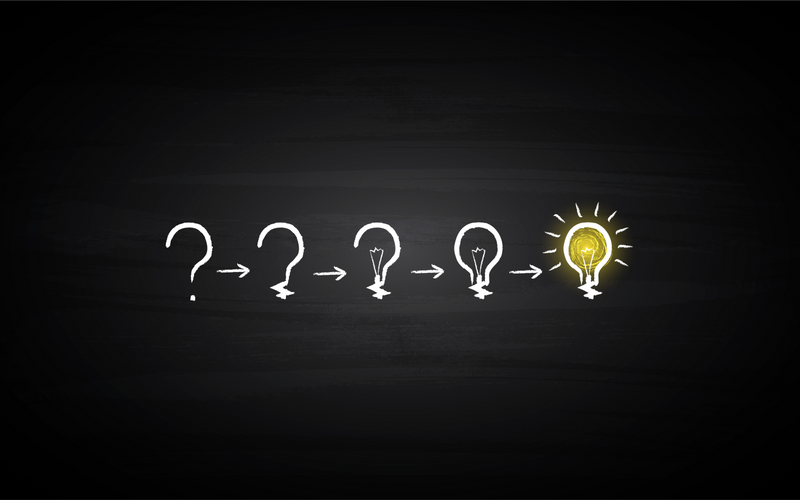
Converting ethical AI principles int practice
11 May 2020
Our Chief Scientist, Tiberio Caetano, has summarised some lessons we have learned over the last year creating practical implementations of AI systems from ethical AI principles. Tiberio is a member of the OECD’s Network of Experts in Artifical Intelligence and wrote this article for the network’s blog.
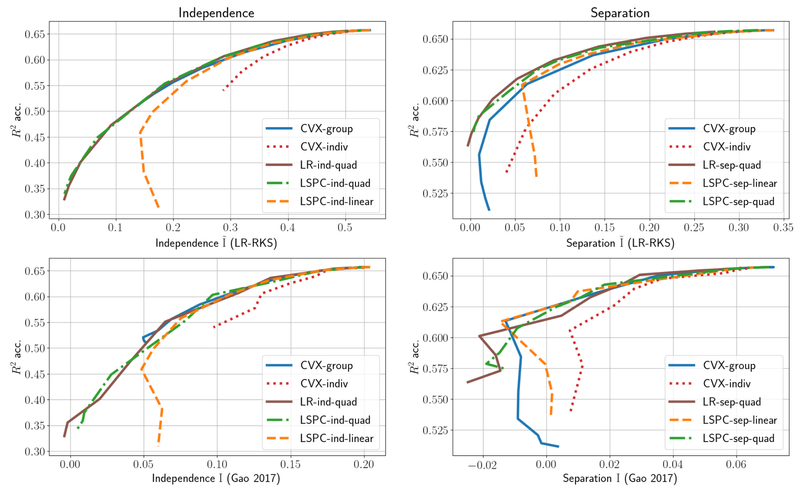
Fast methods for fair regression
25 February 2020
Gradient Institute has written a paper that extends the work we submitted to the 2020 Ethics of Data Science Conference on fair regression in a number of ways. First, the methods introduced in the earlier paper for quantifying the fairness of continuous decisions are benchmarked against “gold standard” (but typically intractable) techniques in order to test their efficacy. The paper also adapts...
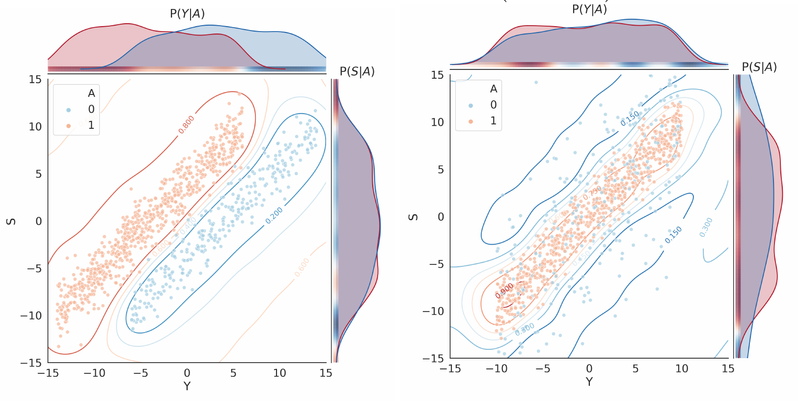
Using probabilistic classification to measure fairness for regression
18 February 2020
Gradient Institute have released a paper (to be presented at the 2020 Ethics of Data Science Conference) studying the problem of how to create quantitative, mathematical representations of fairness that can be incorporated into AI systems to promote fair AI-driven decisions.
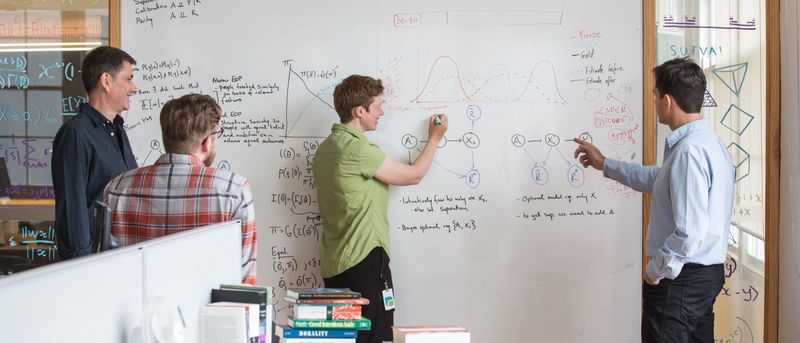
Causal inference with Bayes rule
13 December 2019
Finn Lattimore, a Gradient Principal Researcher, has published her work on developing a Bayesian approach to inferring the impact of interventions or actions. The work, done jointly with David Rohde (Criteo AI Lab), shows that representing causality within a standard Bayesian approach softens the boundary between tractable and impossible queries and opens up potential new approaches to causal...
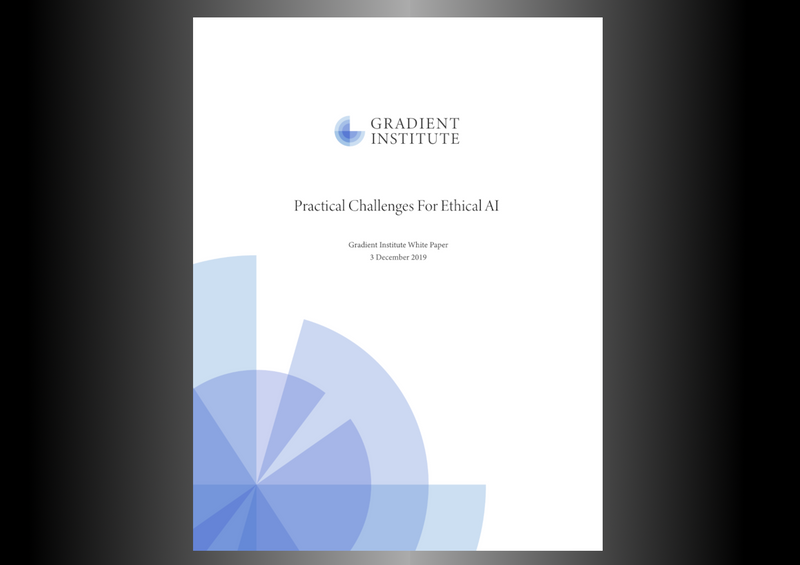
Practical challenges for ethical AI (White Paper)
3 December 2019
Gradient has released a White Paper examining four key challenges that must be addressed to make progress towards developing ethical artificial intelligence (AI) systems. These challenges arise from the way existing AI systems reason and make decisions. Unlike humans, AI systems only consider the objectives, data and constraints explicitly provided by their designers and operators.
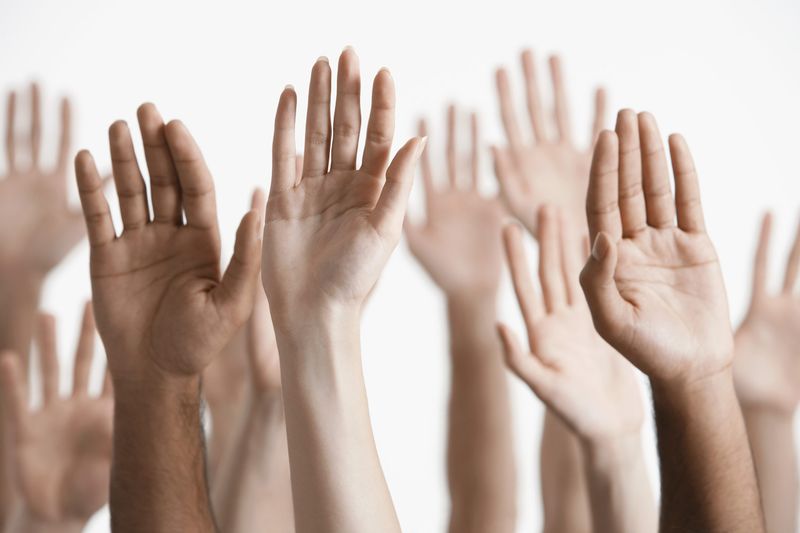
Whose ethics?
25 March 2019
We at the Gradient Institute are often asked who decides the particular ethical stance encoded into an ethical AI system. In particular, because we work on building such systems, the question also takes the form of “whose ethics” we will encode into them. Our Chief Practitioner, Lachlan McCalman, has written a blog post to address such questions.
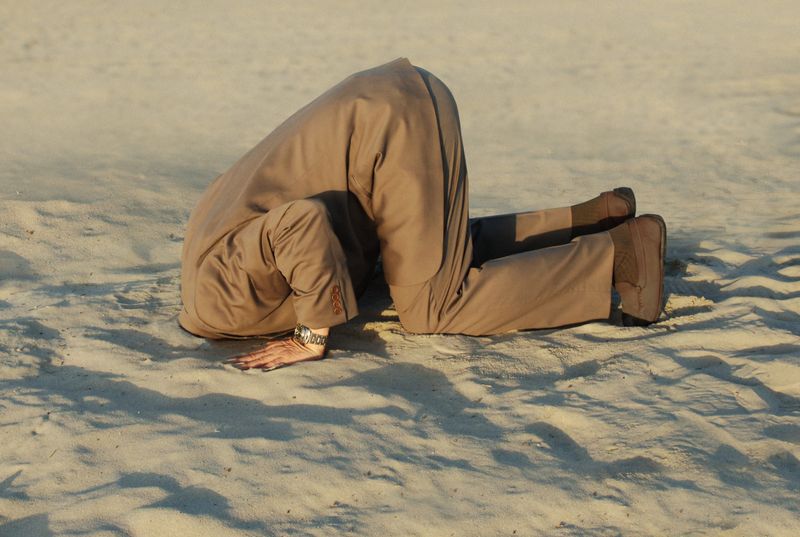
Ignorance isn't bliss
6 December 2018
Societies are increasingly, and legitimately, concerned that automated decisions based on historical data can lead to unfair outcomes for disadvantaged groups. One of the most common pathways to unintended discrimination by AI systems is that they perpetuate historical and societal biases when trained on historical data. Two of our Principal Researchers, Simon O’Callaghan and Alistair Reid,...
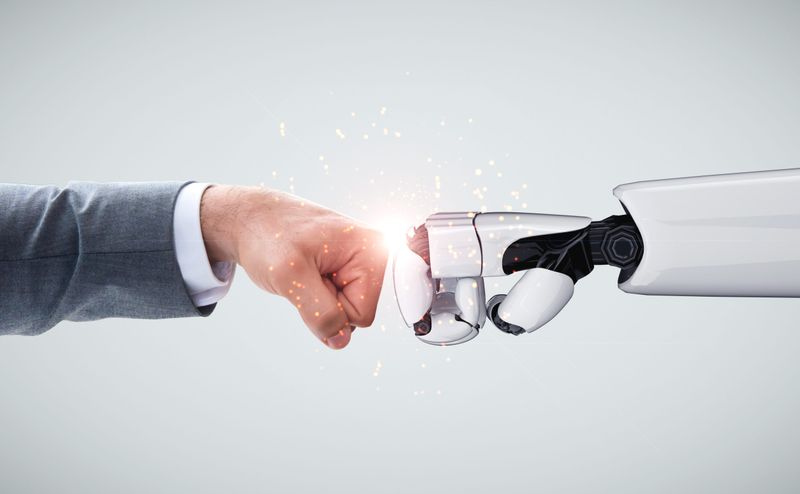
Helping machines to help us
6 December 2018
Societies are increasingly, and legitimately, concerned that automated decisions based on historical data can lead to unfair outcomes for disadvantaged groups. One of the most common pathways to unintended discrimination by AI systems is that they perpetuate historical and societal biases when trained on historical data. Two of our Principal Researchers, Simon O’Callaghan and Alistair Reid,...